
Permute python license#
See the file LICENSE for information on the history of this software, terms & conditions. Do this once to add pkg to Python path License information. Contribute to statlab/permute development by creating an account on GitHub. Plt.subplot(int(bz**0.5),int(np.ceil(bz/int(bz**0. Contribute to statlab/permute development by creating an account on GitHub. Raise Exception("unsupported type! "+str(img.size()))
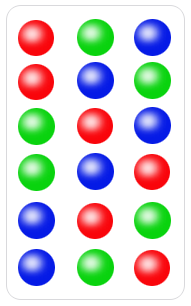
Raise Exception("unsupported type! " + str(img.size())) Print('warning: more than 3 channels! only channels 0,1,2 are preserved!')Įlif bz > 1 and c = 1: # multiple grayscale imagesĮlif bz > 1 and c = 3: # multiple RGB imagesĮlif bz > 1 and c > 3: # multiple feature maps It will return a tensor with the new shape. view() vs reshape() and transpose() view() vs transpose() Both view() and reshape() can be used to change the size or shape of tensors. axisint, optional The axis which x is shuffled along. If x is an array, make a copy and shuffle the elements randomly. Parameters: xint or arraylike If x is an integer, randomly permute np.arange (x). If bz=1 and c=1: # single grayscale imageĮlif bz=1 and c > 3: # multiple feature maps Here, I would like to talk about view() vs reshape(), transpose() vs permute(). method (x, axis0) Randomly permute a sequence, or return a permuted range. Show(x,y,z) produces three windows, displaying x, y, z respectively, where x,y,z can be in any form described above. If x is a 2D tensor, it will be shown as grayscale map
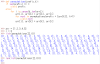
dims (tuple of python:int) The desired ordering of dimensions. If x is a 3D tensor, this function shows first 3 channels at most (in RGB format) permute (input, dims) Tensor ¶ Returns a view of the original tensor input with its dimensions permuted. If x is a 4D tensor (like image batch with the size of b(atch)*c(hannel)*h(eight)*w(eight), this function splits x in batch dimension, showing b subplots in total, where each subplot displays first 3 channels (3*h*w) at most. Show(x) gives the visualization of x, where x should be a torch.Tensor

Input imgs can be single or multiple tensor(s), this function uses matplotlib to visualize. The permutation feature importance is defined to be the decrease in a model score when a single feature value is. This is especially useful for non-linear or opaque estimators. I've written a simple function to visualize the pytorch tensor using matplotlib. Permutation feature importance is a model inspection technique that can be used for any fitted estimator when the data is tabular. # If you try to plot image with shape (C, H, W) Tensor_image = tensor_image.view(tensor_image.shape, tensor_image.shape, tensor_image.shape) Print(type(tensor_image), tensor_image.shape) But PyTorch Tensors ("Image tensors") are channel first, so to use them with matplotlib you need to reshape it: In swap() method, it takes two parameters both are pointers to a character whose values are swapped latter and to reflect this change on the actual parameters we used pointers which help to use the call by reference concept.As you can see matplotlib works fine even without conversion to numpy array. I had made two methods in this program one is two swap the characters passed and other is the recursive method.

I had used the concept of recursion and also used the pointers. In this project, you will be asked to give your name and then it will print all the possible arrangements of your name and also print the total number of ways. I had made this project on permutation in which not only it will print the total number of ways but also print all the arrangements also. A permutation is a mathematical concept in which it gives the total number of ways a set can be arranged.Ī set can be of numbers or characters.
